MSU-led international research team receives $1 million grant to build multi-disciplinary precision livestock farming network
Building a multi-disciplinary livestock farming network is the focus of a new $1 million grant from the U.S. Department of Agriculture’s Data Science for Food and Agricultural Systems program.
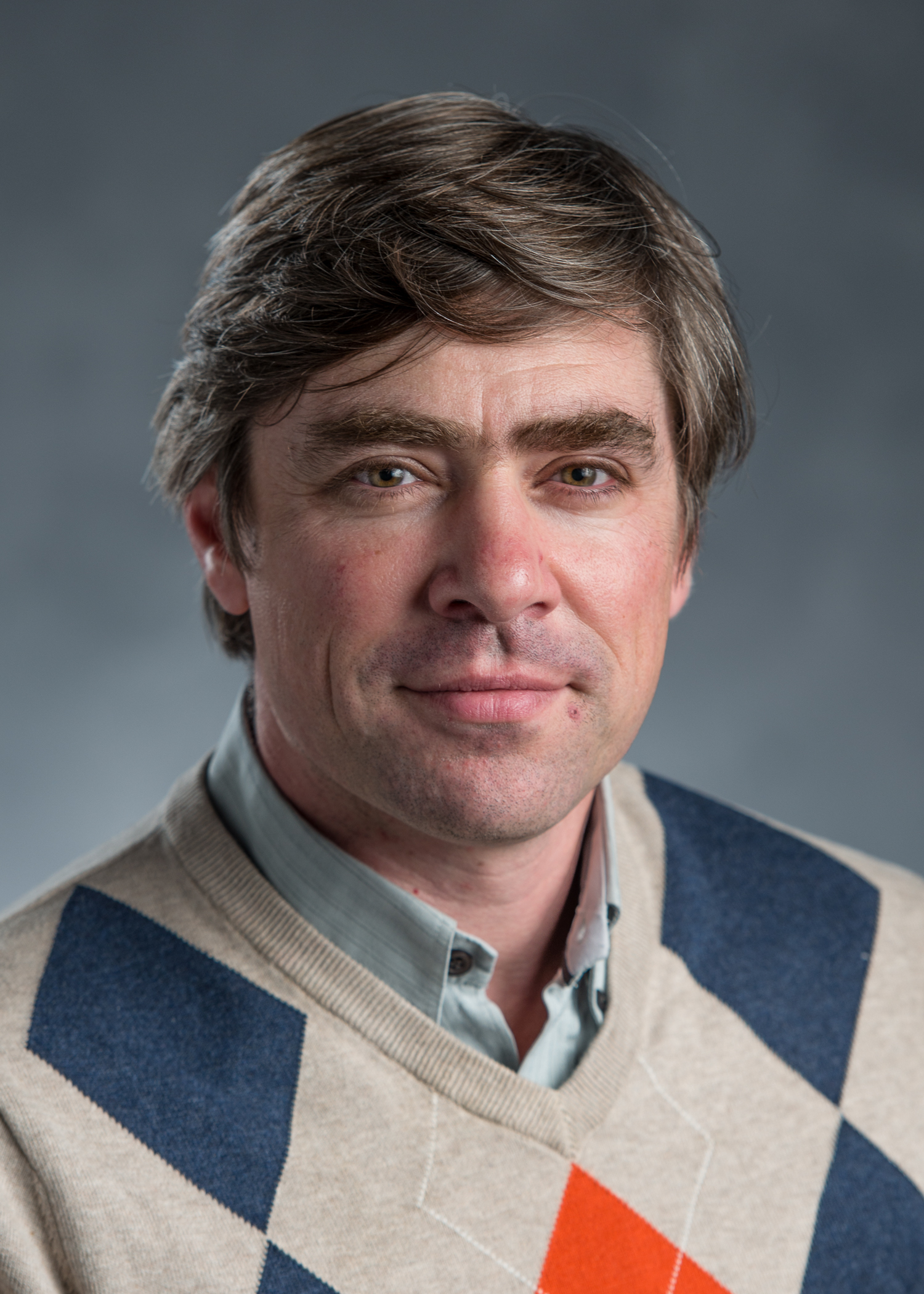
EAST LANSING, Mich. — A team of international researchers led by Michigan State University has received a five-year, $1 million grant from the U.S. Department of Agriculture’s (USDA) Data Science for Food and Agricultural Systems (DSFAS) program, a component of the National Institute of Food and Agriculture.
One of the main priorities of the DSFAS program is the formation of Coordinated Innovation Networks that solve critical problems in food and agriculture production. The newly funded, MSU-led project is aimed at establishing a multidisciplinary network of researchers in animal science, computer science and engineering to improve computer vision systems in precision livestock farming. Ideally, these powerful computer vision systems allow producers to make real-time management decisions at the individual animal level, thus increasing productivity and profits.
Juan Steibel, an associate professor in the MSU departments of Animal Science and Fisheries and Wildlife, is the principal investigator.
“Due to the costs of technology, widespread implementation of precision livestock farming practices hasn’t occurred,” he said. “There is a significant need for the costs to be lowered, so there is room for innovation.”
Also on the project from MSU are Janice Siegford, a professor in the Department of Animal Science; Madonna Benjamin, an assistant professor and swine extension veterinarian in the MSU College of Veterinary Medicine and MSU Extension; and Daniel Morris, an associate professor in the College of Engineering.
Steibel said that one of the primary goals is to recruit passionate computer scientists and engineers to join precision livestock farming efforts, which is needed to improve the quality of data collection.
Pigs and cattle will be the focus of the project, and both new and existing data will be analyzed. To collect new information, researchers at MSU will utilize the on-campus Swine Teaching and Research Facility, which operates like a small-scale swine farm.
Guilherme Rosa and Joao Dorea, scientists at the University of Wisconsin-Madison, will use an on-campus dairy research farm, while Tami Brown-Brandl and Eric Psota, researchers at the University of Nebraska-Lincoln, will operate at the USDA’s Meat Animal Research Center in Clay Center, Nebraska.
“Computer vision can really improve livestock farming systems by enhancing animal welfare, monitoring animal behavior, detecting illnesses earlier, and by allowing the assessment and measurement of many animal parameters that are either very difficult or even impossible to measure without the utilization of cameras and computer algorithms,” Rosa said. “Basically, anything we do today using visually subjective assessment can be done better with computer vision.”
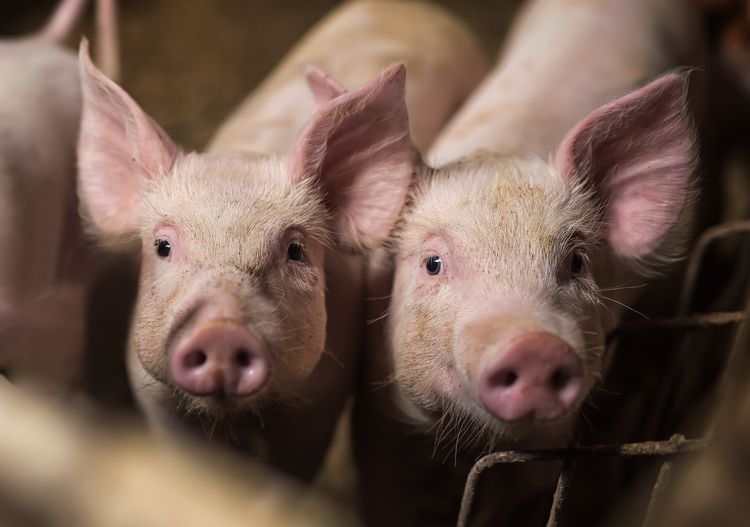
At each of the research locations, cameras will be strategically positioned around the farms, and animal scientists will write annotations that describe what the images capture. The information will then be analyzed by computer scientists to create algorithms that identify and track animals, and to determine what activity they are engaged in. Once that occurs, strengths and weaknesses of the analysis can be determined.
“If an image showed the head of a pig near another pig’s body, for example, we need to know what’s actually happening,” Steibel said. “This could be an aggressive behavior such as biting, or it could be grooming. This is where humans have to be accurate to make the computer vision program as powerful as possible.”
Tomas Norton, an assistant professor at the Catholic University of Leuven in Belgium, will assist on the engineering side. He has collaborated with multiple members of the team on previous projects, using technology to monitor animal behavior.
The team will make the images and datasets publicly available, and articulate deficiencies in the current computer vision algorithms. Steibel said that ideas will be solicited from computer scientists and engineers on how to solve computer vision problems and from animal scientists on contributing well-annotated images and production data. Public forums and webinars will be scheduled to discuss the challenges and the findings as well.
“We’re hoping that researchers across disciplines are compelled to join us in addressing the issues we present,” Steibel said. “The more diverse our team is in knowledge and experience, the better chance we have to come up with novel solutions.”